The integration of AI into scientific research has become an exciting development in recent years. As scientists explore new frontiers, AI discovery systems, especially large language models (LLMs), have proven useful for identifying novel concepts and patterns. However, despite their potential, these systems often only address the “easy problems” of science, while the more complex challenges remain unsolved.
The "Easy Problem" vs. The "Hard Problem"
AI has already made impressive strides in areas like protein folding, where tools like AlphaFold 2 have changed the landscape of biology. These systems are incredibly good at solving problems once the parameters are well-defined and understood. Scientists provide the AI with a clear representation of the problem, and the AI executes solutions based on pre-established data. This is what researchers call the "easy problem."
The easy problem isn’t without its challenges—it requires extensive data and advanced algorithms. But the reason it's considered "easy" is that the problem is already well-defined, with clear goals and boundaries. AI excels at optimizing these known parameters. It can refine, interpret, and predict within the scope of provided data. However, the role of AI in defining the problem itself is where things get tricky.
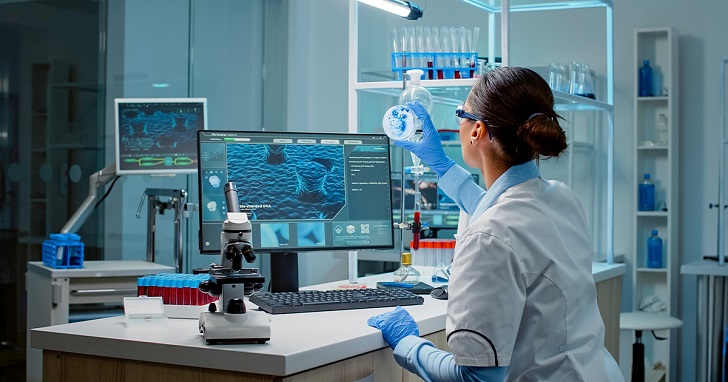
Rulex | Scientists provide the AI with a clear representation of the problem, and the AI executes solutions based on pre-established data.
AI Discovery and Its Limitations
The challenge lies in AI’s ability—or inability—to solve the "hard problem." This hard problem refers to AI’s current limitations in generating entirely new scientific questions or defining its problem space. Human researchers evolve the scope of a problem through observation, reflection, and hypothesis testing. AI, on the other hand, relies on human input to define the parameters within which it works. It cannot independently formulate entirely new scientific questions.
AI systems work well when humans provide well-structured datasets and clear objectives. However, the process of discovering new, unknown scientific problems involves creativity, intuition, and trial and error—qualities that AI lacks. Therefore, while AI can optimize and improve on known issues, it cannot independently uncover unknown frontiers of science.
Human Input in AI Discovery
Even the most advanced AI systems depend on human input to set the parameters and constraints that guide their problem-solving process. Human scientists bring creativity, intuition, and interdisciplinary thinking to the table, which AI currently cannot replicate. In every scientific breakthrough, the problem evolves alongside new discoveries, with scientists continuously reassessing and refining their approach.
Human involvement is critical for guiding AI systems toward meaningful results. AI tools may be excellent at interpolating data and even predicting trends based on known datasets. However, the act of uncovering a completely new problem—something no one has thought to ask—is a task that only human intuition can handle. AI operates within the space defined by human scientists, and this limitation is significant when it comes to tackling the most complex scientific challenges.
The Role of Constraints in AI Success
For AI to function effectively in research, the constraints within which it operates must be carefully defined. These constraints often determine the success of AI discovery systems. For example, tools like AlphaFold 2 can make predictions within the defined parameters of protein folding, but it cannot venture beyond those boundaries to ask new questions about biology.
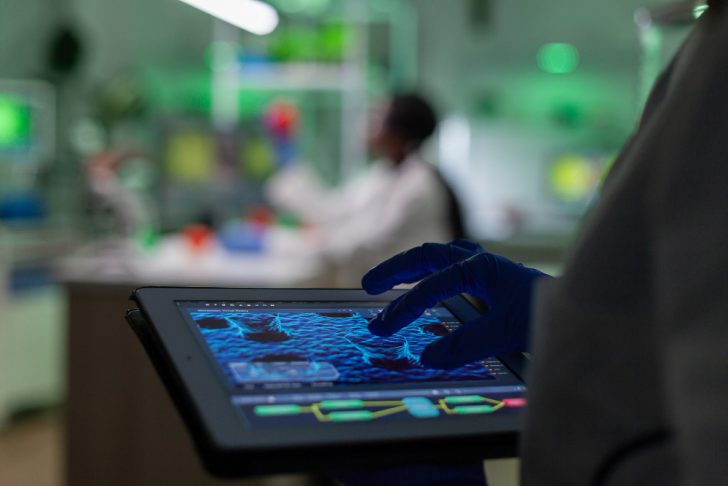
DC Studio | Freepik | AI requires guidance and structure from humans to achieve meaningful outcomes.
Setting the right constraints for AI ensures that the system functions optimally, but it also highlights the need for human creativity in scientific research. AI requires guidance and structure from humans to achieve meaningful outcomes. Without clearly defined goals, AI systems can only extrapolate from existing data, not generate entirely new questions or solutions.
The Future of AI Discovery
As scientists continue to integrate AI into their research, the goal is to develop systems that can tackle more complex scientific problems. This means not just optimizing known data but also contributing to the generation of new scientific questions. Some researchers believe that understanding the cognitive science behind human problem-solving could lead to breakthroughs in AI discovery.
By studying how human scientists think and solve problems, researchers may one day create AI systems that act like research assistants. These AI systems would require natural language instructions and ongoing guidance from experts. However, this also raises questions about the social and collaborative nature of science itself.